Carleton University - School of Computer Science Honours Project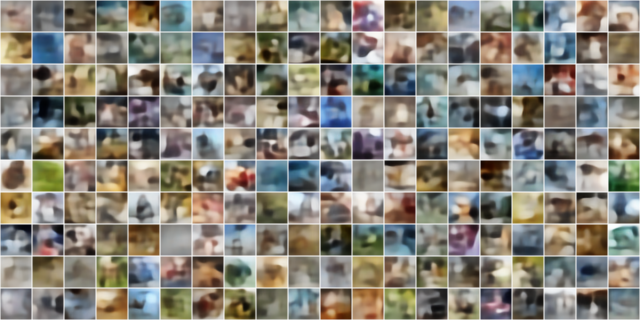
Winter 2018
Zero-Shot Learning by Bridging Prediction and Explanation
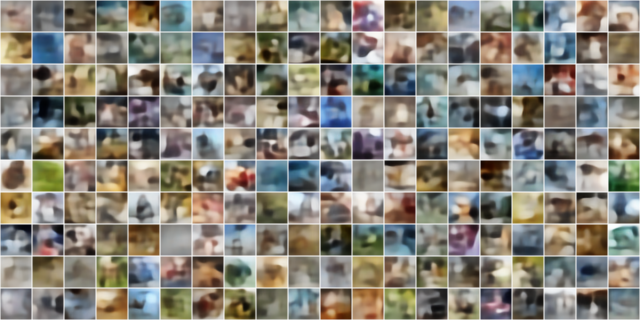
ABSTRACT
Human learning is distinguished for its efficiency. Compared to the most successful learning algorithms, we learn much richer representations and from far fewer examples. Newly learned concepts are comprised by a structure which overlaps with a lifetime of prior experience, allowing us to build rich conceptual models that enable a variety of functions for free. Several works argue that current approaches to computational intelligence are focused on solving the wrong problem, that being one of solely pattern recognition, and that our flexible learning capabilities are made possible by building causal models of our world, where compositionality and learning-to-learn are crucial for generalizing knowledge to novel tasks. Motivated by this, we approach the problem of zero-shot visual recognition. We explore a recurrent variational-autoencoding framework equipped with a spatial attention mechanism which we believe can learn to approximate causal information about the domain it models. We then define a nonlinear learning compatibility framework between this causal information and images in order to perform zero-shot visual recognition.