Carleton University - School of Computer Science Honours Project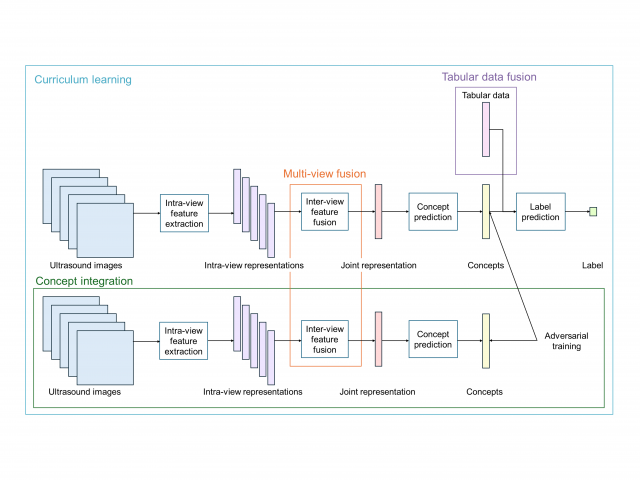
Winter 2024
Integrating Domain Knowledge in Deep Learning for Pediatric Appendicitis Diagnosis
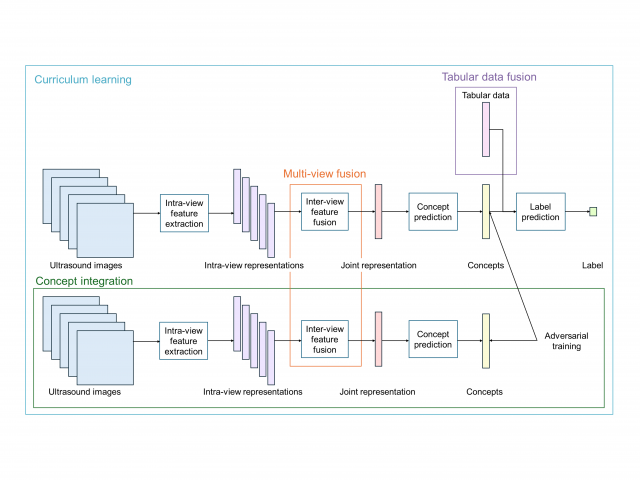
ABSTRACT
Disease diagnosis through medical imaging data remains challenging due to the inherent heterogeneity in disease manifestations and visual representations. In this work, we introduce domain knowledge-guided deep learning approaches for predicting the diagnosis, severity, and management plans. Our model integrates ultrasound imaging with patient records and test results, including demographic, clinical and laboratory data, to comprehensively capture diagnostic information. We further enhance feature fusion in multi-view images using the self-attention mechanism, effectively identifying correlations between unordered views and managing information overlap. Next, by guiding representation learning with expert-defined concepts, we improve the interpretability and performance of diagnostics. Moreover, we adopt curriculum learning to progressively train our model on more complex targets, mimicking the medical training pattern to improve its predictive performance. We compute results on a pediatric appendicitis dataset. Results show that incorporating patient records and test results improves the areas under receiver operating characteristic (AUROC) and precision-recall (AUPR) curves by 0.15 and 0.11, respectively. Self-attention for feature aggregation outperforms long short-term memory based aggregation, enhancing both AUROC and AUPR by 0.04 on average across three predictive tasks. Further, applying concept-guided multi-task learning improves the AUROC by 0.02 and AUPR by 0.04 in the management task. Applying curriculum learning to severity and management tasks achieves average increases of 0.03 in AUROC and 0.07 in AUPR. In summary, incorporating domain knowledge into imaging tasks has value, although different types of domain knowledge improve performance to different extents.