Carleton University - School of Computer Science Honours Project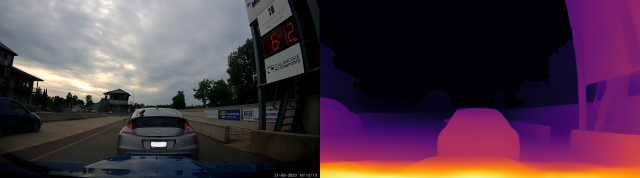
Winter 2024
Transforming Monocular Video into 3D Environments: Challenges, Innovations, and Future Directions
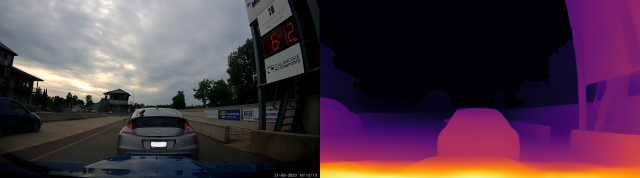
ABSTRACT
Monocular depth estimation and 3D reconstruction from video have significant potential in applications such as autonomous driving, robotics, and augmented reality. However, existing approaches face challenges in handling diverse environments, dynamic objects, and the lack of representative datasets. This thesis presents a comprehensive review of the current state of monocular depth estimation and 3D reconstruction, identifying key areas for improvement and proposing a robust framework for transforming monocular video into 3D environments. The proposed approach leverages attention mechanisms at different scales, incorporates masked token prediction for temporal reasoning, and integrates static/dynamic discrimination for handling moving objects. The thesis addresses the need for diverse datasets by proposing synthetic data generation techniques and domain adaptation methods. Although the framework was not implemented due to time constraints, the conceptual approach provides a promising direction for future research. The potential impact extends to various applications where robust and accessible 3D mapping is crucial. The thesis discusses challenges and future research directions, including efficient architectures, collaborative mapping, and integration with embodied AI systems. This work contributes to the advancement of monocular depth estimation and 3D reconstruction by providing a comprehensive review and proposing a novel end-to-end framework for transforming monocular video into 3D environments.